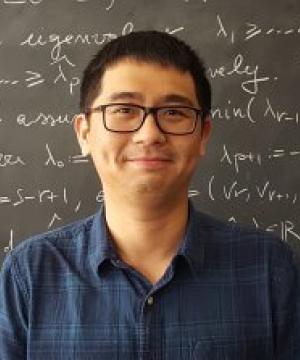
Abstract: Collective behaviors (also known as self-organization) can be found in studying superconductivity, crystal formation, aggregation of cells/animals, social behaviors of insects and humans, consensus of opinions, etc. It is a challenging task to understand these behaviors from the mathematical point of view. We offer a statistical/machine learning approach to understand such behaviors from observation data; moreover, our learning approach can aid in validating and improving the modeling of collective behaviors.
We develop a learning framework to derive interpretable models to explain the observation data using dynamical systems. We provide a convergence theory in terms of the number of different initial conditions for first-order systems of homogeneous agents, and investigate its performance for various first- and second-order systems of heterogeneous agents. We then study the stead state properties of our learned models, and extend the learning framework to include more complicated structures. We complete the convergence analysis of second-order systems, and extend the approach to learn dynamical models for agents constrained on Riemannian manifolds.
Having successfully applied our learning method to various set of simulated dynamical data, we then try it on the National Aeronautics and Space Administration Jet Propulsion Laboratory’s modern Ephemerides (trajectory data of the celestial bodies in the Solar system). We find that our learned model can outperform the Newton's model (based on Newton's universal law of gravitation) in terms of reproducing the position/velocity of major celestial bodies, as well as preserving geometric properties (period/aphelion/perihelion) of the trajectory and highly-localized perihelion precession rates of Mars, Mercury, and the Moon. Upon careful inspection of our model, we discover that it even captures potion of the general relativity effects.
Speaker’s Bio: Ming Zhong is currently working as a postdoctoral data scientist in the Texas A&M Institute of Data Science (TAMIDS) at Texas A&M University. Before his position at Texas A&M, he was a postdoc fellow working with Mauro Maggioni at Johns Hopkins University. He obtained his Ph.D. in Applied Math from University of Maryland under the guidance of Eitan Tadmor.
Last Updated: August 12, 2021 - 12:21 pm