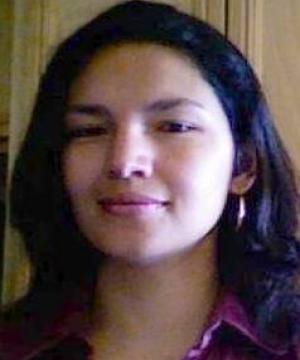
Abstract: Identifying noisy samples and outliers at training time is crucial to achieving good performance and improving robustness of deep learning models. However, this process can be difficult and excessively time-consuming if attempted by hand, especially for large data sets. Moreover, in realistic situations, there are gradations in our judgment about noisy samples. In this work, we present a deep abstaining regressor (DAR) model and an extension of the Deep Abstention Classifier (DAC) framework to regression problems. We apply the new DAR formulation to synthetic and real data sets and demonstrate that the model is able to identify noisy samples, reducing their influence during training while learning to quantify the uncertainty in the predictions via a heteroscedastic criterion. DAR constitutes a new tool for the machine learning practitioner, producing actionable uncertainty quantification predictions and helping with the automatic curation of data
Speaker’s Bio: Cristina Garcia-Cardona received a B.Sc. degree in electrical engineering from Universidad de Los Andes, Colombia, and a Ph.D. degree in computational science from Claremont Graduate University and San Diego State University Joint Program, CA, USA. She is currently a Staff Scientist with the Computer, Computational, and Statistical Sciences (CCS) Division, at Los Alamos National Laboratory. Her research interests include deep learning models for inverse problems in imaging, uncertainty quantification for regression problems, reinforcement learning, and sparse representations.
Last Updated: October 30, 2023 - 7:47 am