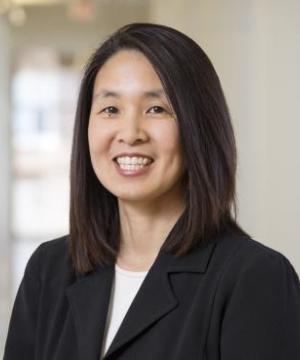
Abstract: Researchers in all disciplines desire to identify causal relationships. Randomized experimental designs isolate the treatment effect and thus permit causal inferences. However, experiments are often prohibitive because resources may be unavailable, or the research question may not lend itself to an experimental design. In these cases, a researcher is relegated to analyzing observational data. To make causal inferences from observational data, one must adjust the data so that they resemble data that might have emerged from an experiment. Traditionally, this adjustment has relied on statistical models such as the propensity score models despite the fact that, in the 40 years since the idea was introduced, we have not advanced in our ability to correctly specify a parametric model for treatment propensity. We propose a model based on a framework that moves away from a statistical modeling framework tied to individual matched units toward a machine learning framework aimed at creating statistically indistinguishable treatment and control groups within the framework of a generative adversarial model.
Speaker’s Bio: Wendy K. Tam Cho is Professor in the Departments of Political Science, Statistics, Mathematics, Computer Science, Asian American Studies, and the College of Law, Senior Research Scientist at the National Center for Supercomputing Applications; Faculty in the Illinois Informatics Institute; and Affiliate of the Cline Center for Advanced Social Research, the Computational Science and Engineering Program, and the Program on Law, Behavior, and Social Science at the University of Illinois at Urbana-Champaign. She is also a Fellow of the John Simon Guggenheim Memorial Foundation and the Society for Political Methodology.
Last Updated: October 13, 2023 - 10:36 am