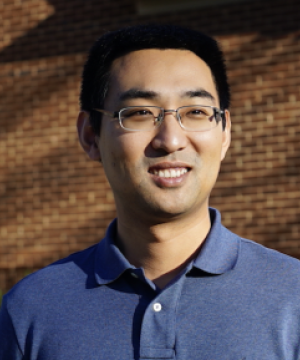
Abstract: In this work, we introduce a stochastic maximum principle (SMP) approach for solving the reinforcement learning problem with the assumption that the unknowns in the environment can be parameterized based on physics knowledge. For the development of numerical algorithms, we apply an effective online parameter estimation method as our exploration technique to estimate the environment parameter during the training procedure, and the exploitation for the optimal policy is achieved by an efficient backward action learning method for policy improvement under the SMP framework. Numerical experiments are presented to demonstrate that the SMP approach for reinforcement learning can produce reliable control policy, and the gradient descent type optimization in the SMP solver requires fewer training episodes compared with the standard dynamic programming principle-based methods.
Speaker’s Bio: Feng Bao is an Associate Professor and the Timothy Gannon Endowed Professor of Mathematics at Florida State University. Prior to his appointment at Florida State University, Feng was an Assistant Professor of Mathematics at the University of Tennessee at Chattanooga, and he spent two years as a Postdoc at Oak Ridge National Laboratory. Feng's research lies in applied and computational mathematics with a focus on stochastic optimization and stochastic optimal control, mathematics for machine learning, scientific machine learning, data assimilation and inference for stochastic processes, uncertainty quantification, analysis and numerical solutions for stochastic differential equations, analysis and numerical solutions for stochastic partial differential equations.
Last Updated: October 31, 2023 - 3:18 pm