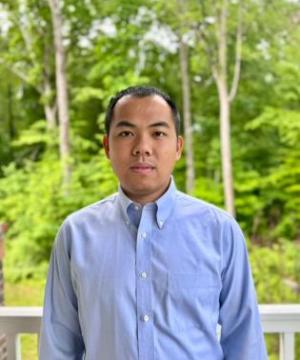
Abstract: In this talk, I will introduce a novel score-based generative sampling framework to solve the high-dimensional nonlinear filtering problem. Traditional methods such as particle filters and ensemble Kalman filters often suffer from low accuracy or instability in this high-dimensional nonlinear scenario.
Our approach integrates a score-based diffusion model within the recursive Bayesian filtering framework, resulting in the development of the Score-based Filter (SF). The key idea to the SF is the use of the score representation to store the recursively updated filtering distribution, rather than maintaining the filtering distribution itself. By leveraging the reverse-time diffusion process, SF can generate unlimited samples to characterize the filtering density. An essential aspect of SF is its analytical Batesian update step, gradually incorporating data information into the score representation. This analytical inclusion of data likelihood is pivotal for addressing the degeneration issues prevalent in very high-dimensional nonlinear filtering challenges. We demonstrate the efficacy of SF through three benchmark problems, including the 100-dimensional stochastic Lorenz system, a notoriously difficult challenge for existing methods.
Additionally, I will present the Ensemble Score Filter (EnSF), developed to address challenges in even higher dimensions. EnSF overcomes the issue of high dimensionality by utilizing a training-free score estimation method that employs a mini-batch-based Monte Carlo estimator. This estimator directly approximates the score function at any spatial-temporal point, in contrast to traditional diffusion models that rely on neural networks for score approximation. The advantage of this training-free approach is twofold: it maintains sufficient accuracy for high-dimensional nonlinear problems and significantly reduces the time required for training neural networks. We demonstrate the effectiveness of EnSF using high-dimensional Lorenz systems, where it consistently achieves impressive results in accurately tracking extremely high-dimensional systems (up to 1,000,000 dimensions) with complex nonlinear observation processes—a notable challenge for existing filtering methods.
Speaker’s Bio: Zezhong (Neil) Zhang is currently a post-doc in the Data Analysis and Machine Learning group. He received his Ph.D. in Financial Mathematics from Florida State University with emphasis in probabilistic machine learning and nonlinear filtering in 2023. Before this, he obtained a master’s in quantitative finance and risk analytics at Rensselaer Polytechnic Institute, Troy, New York, and a bachelor’s degree in financial engineering from Southwestern University of Finance and Economics, Chengdu, China.
Last Updated: May 9, 2024 - 8:38 am