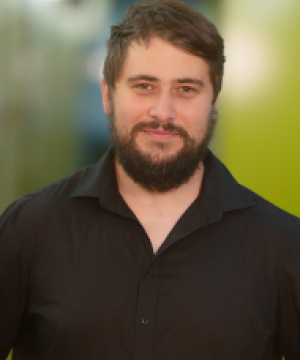
Abstract: Systems of ordinary differential equations are an established tool for modeling a wide range of complex processes across all disciplines. In many instances, the behavior of the quantity resembled by the state dynamics can be influenced indirectly by choosing a suitable control input. Often, this needs to be done in an optimal way, i.e., by minimizing an energy functional, leading to a mathematical program with ordinary differential equation constraints.
In this context, particular interest lies in feedback controls, i.e., controls that are constructed as a function of the state variable at every point in time. However, computing an optimal feedback law requires the solution of a Hamilton-Jacobi-Bellman equation, which already becomes computationally intractable for moderate dimensions.
In this talk, a learning approach for optimal feedback gains for nonlinear continuous time control systems is proposed and analyzed. The goal is to establish a rigorous framework for computing approximating optimal feedback gains using universal approximators, e.g. neural networks or polynomials. The approach rests on two main ingredients. First, an optimal control formulation involving an ensemble of trajectories with 'control' variables given by the feedback gain functions. Second, an approximation to the feedback functions by a parametrized family of universal approximators. Based on universal approximation properties we prove the existence and convergence of optimal feedback controllers. The talk is accompanied by numerical examples, e.g. an optimal consensus problem.
Speaker’s Bio: Daniel Walter is Assistant Professor for Nonsmooth Optimization at the Humboldt Universität zu Berlin. He received his Ph.D. from the Technical University of Munich in 2019 and held a Postdoc position at the Johann Radon Institute for Computational and Applied Mathematics in Linz until 2022. His work primarily focuses on the design and analysis of efficient solution algorithms for nonsmooth minimization problems in infinite dimensional function spaces, as well as on the interplay between optimal feedback control and machine learning.
Last Updated: August 23, 2023 - 12:39 pm