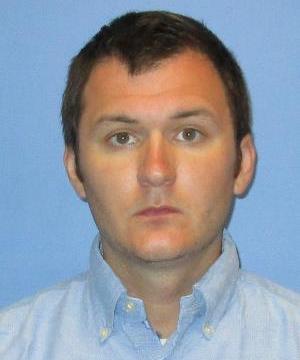
Abstract: Many common data analysis tools (for example, the fast Fourier transform) assume a particular structure of their input, are so are unsuited to general point set data. The analysis of such data often begins with the discovery or imposition of some underlying structure, which then serves as the basis for regression algorithms, visualization routines, etc. Hierarchical clustering algorithms, which impose a tree structure on their input, are commonly used for this step. The main topic of this talk is a technique for the decomposition and compression of point set data organized into a hierarchical clustering tree. We derive error bounds on the compression loss, consider the cost of storing the compressed tree along with the compressed data, and, in the case of Euclidean point set data, study the relationship between the Euclidean metric and notions of distance on the tree.
Speaker’s Bio: Ben Whitney is a postdoc in the Computational and Applied Mathematics group at the Oak Ridge National Laboratory. He received his PhD in Applied Mathematics from Brown University under the supervision of Mark Ainsworth. After graduating in 2018, he took a postdoc position at Brown and then came to the lab in 2019. He works in compression methods for scientific data and scientific software development.
Last Updated: September 8, 2020 - 11:26 am