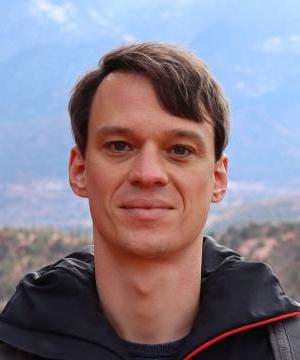
Abstract: The Euler equations with gravity are the foundation of dynamical cores of atmosphere, ocean, and cloud resolving models used in climate and weather prediction. A major challenge arises from the relative speed of acoustic waves, which are often orders of magnitude faster than advective processes (i.e., the Mach number is low). Although many simplifications, such as (pseudo-)incompressible, anelastic, or hydrostatic approximations can be used to eliminate the fast waves, they incur an additional modeling error.
Due to advances in computational power, cloud resolving models can be built on the Euler equations directly, without additional simplifications. However, a direct discretization of the underlying hyperbolic conservation law with finite volumes requires very high order methods and small time steps to overcome high diffusivity and Courant–Friedrichs–Lewy -limits. In order to alleviate this, we investigate implicit-explicit splitting methods that aim to cleanly separate the Euler equations into fast and slow modes. Then, the fast mode is discretized implicitly in time, and the slow mode is treated explicitly, in a way to be asymptotically compatible in the low-Mach limit with a (pseudo-)incompressible model. This mathematical property guarantees that the Mach number has no impact on the time-step requirement and numerical diffusion. We are investigating the behavior of the proposed method in the context of test cases for various regimes of the Euler equations.
Speaker’s Bio: Konstantin Pieper is a staff member in the Data Analytics and Machine Learning group, which he joined in June 2019. He obtained his Ph.D. in Mathematics from the Technical University Munich in 2015 and, prior to joining the Oak Ridge National Laboratory, he worked as a postdoctoral researcher at the Florida State University. His research interests include structure preserving discretization methods in the context of earth system models (atmosphere and ocean), sparse optimization, inverse problems, sensor placement, and machine learning.
Last Updated: June 29, 2023 - 7:30 am